While the concept of employee turnover prediction is far from new, the advent of Artificial Intelligence (AI) has ushered in a new era, providing unprecedented advantages and capabilities for this crucial HR function.
Machine learning, in particular, has revolutionized the way organizations approach employee turnover. By leveraging the vast amount of data at their disposal, businesses can now make more accurate predictions, identify underlying factors, and formulate targeted strategies for employee retention.
In spite of all these advantages, embracing AI to predict employee turnover requires some special considerations not only in terms of the actual tools and know-how you want to bring into your organization but, more importantly, in terms of the framework you need to establish across your business to use this technology effectively.
The long-term trend of high employee attrition
Nobody in the corporate world should ignore the fact that employee turnover has been growing steadily for more than one decade and is expected to continue growing.
According to professors Joseph Fuller and William Ker, the record number of American workers who quit their jobs in 2021, wasn’t an anomaly brought by the pandemic. That number, “included many workers who might otherwise have quit in 2020 had there been no pandemic. We’re now back in line with the pre-pandemic trend, which is one that American employers are likely to be contending with for years to come,” explain Fullen and Ker.
This view is widely shared by many HR experts. “New employee expectations, and the availability of hybrid arrangements, will continue to fuel the rise in attrition,” argues Piers Hudson, senior director in the Gartner HR practice.
Which employee retention strategies can be used to reduce employee turnover?
Companies, of course, are aware of the ongoing attrition in the workplace and the most proactive ones have been addressing this phenomenon with a series of business strategies aimed at fostering employee engagement and retention. Some of this strategies include the following:
- Improve the onboarding and hiring processes
- Offer competitive compensation and benefits
- Provide opportunities for growth and development
- Build a positive work environment
- Offer work-life balance initiatives
Along those lines, companies have been also implementing turnover prediction analyses. From exit interviews and employee surveys to absenteeism tracking and performance evaluations, most organizations are already using people analytics to anticipate employee turnover.
According to The Use of People Analytics in Human Resources report elaborated by SHRM, 82% of organizations use people analytics to assess employee retention and turnover. This significant percentage, however, doesn’t mean that 82 % of organizations are doing it right.
In fact, most companies foresee employee turnover using data from things like exit interviews and annual employee surveys that “don’t give managers a real-time picture of who might be considering leaving,” explain professors Brooks Holtom and David Allen.
Considering these kinds of limitations as well as the ability that AI models offer to handle large volumes of data, improve accuracy and real-time analysis, provide tailored insights, and learn and adapt on a continuous basis, more and more companies are embracing AI-driven technology to identify people at risk of leaving their organizations.
Top 5 best practices for using AI to predict employee turnover
As we mentioned at the beginning of this article, there are some special considerations you need to keep in mind if you want to incorporate AI into your employee turnover analyses. Let’s take a look at some of the most crucial ones.
1. Build your own infrastructure and train your workforce
You may be tempted to buy a third-party software to measure employee attrition across your organization. However, you will still need a solid infrastructure and people with the necessary know-how to use AI models across your business.
“Before driving onto the AI express lane, first invest in the necessary infrastructure, data literacy, and technology to use people analytics effectively and appropriately,” recommends the SHRM report.
If you want to start building an AI-driven friendly environment for your organization, you should consider reskilling and upskilling your workforce in data literacy and people analytics fundamentals.
2. Make sure you have good quality data
Having an efficient and advanced machine learning system in place will help with your attrition analyses. However, if the data is irrelevant and disconnected, not even the most advanced AI system will be able to give you valuable insights.
According to the SHRM report, “a comprehensive audit of your organization’s data infrastructure, training, resources, governance systems, and procedures is a top priority if your organization is seeking to advance its people analytics capabilities.”
The following are some of the actions that will help you to have good quality data:
- Standardize data collection using consistent data entry methods, formats, and naming conventions.
- Validate and verify data to minimize errors and inconsistencies.
- Clean and preprocess regularly your data to remove duplicates, correct errors, and handle missing values.
- Analyze historical data to identify patterns and trends in employee turnover.
- Enhance your dataset by incorporating additional relevant data sources.
3. Use turnover predictions to leverage your talent management strategy
Anticipating your turnover rate is something you do in order to improve your overall talent management strategy. In other words, foreseeing employee turnover must be part of a broader strategy aimed at improving your employee retention and engagement.
“Because of all the different factors that affect turnover, it’s important to look at your resignation metrics in-depth so you can focus on the right areas and not just to see what happened, but understand why it happened, what will happen next, and how to adapt your retention strategy to align with company objectives,” explains Zack Johnson, GM, Embedded Analytics & Mid-Market at Visier.
Having an accurate system for Identifying people at risk of leaving your organization doesn’t mean anything if you don’t use that information to improve your retention strategy and have meaningful conversations with those key employees you don’t want to lose.
4. Be transparent to build trust and tackle privacy concerns
Being open and transparent about the data you want to collect, how you are planning to use it, and what benefits the whole process brings to your employees is the best way to remove privacy concerns among your workforce.
Furthermore, if you are able to build trust across your organization, you will be able to reinforce your retention and engagement strategies. “Trust and retention often go hand in hand, so use your data responsibly to build a happier, more transparent workplace,” advises Samantha McLaren, lead copywriter of Global Impact at LinkedIn.
Similarly, Bill Nowacki, decision science lead at KPMG, suggests building a process that is focused on the benefits to the employees and allows them to opt in into the system voluntarily. “We can spot you burning out before you notice you’re burning out. When you present it that way, that we’re looking out for your health, they opt in,” argues Nowacki.
Professor Allen also suggests “building trust in the systems which means being thoughtful about where to insert humans in the decision making process and maintaining human oversight of data and its use.”
Apart from all that, you should also adhere to data privacy regulations (e.g. GDPR, HIPAA), protect sensitive employee information, anonymize data when necessary, and document data collection to maintain transparency and consistency.
5. Use AI models ethically
AI models aren’t entirely bias-free. Because of that you need to understand the bias in the system. “The ethical responsibility for us as leaders has to be that we have to understand what is biased today in our systems. How representative is your data? How representative is your training? How representative is your labeling? Those are essential, essential questions that need to be part of the executive conversation,” argues Karim Lakhani, professor at Harvard Business School.
If there’s something that you have to learn about machine learning is that your understanding of how these models are built for you needs to be constant. “That’s where the learning mandate doesn’t stop, because you have to understand how these machine learning systems are built for you, to understand what the biases are, and how you might get sued or be put in jail, for God’s sakes, if you don’t follow through on these things,” highlights Lakhani.
To avoid these kinds of risks, make sure to do the following with your data:
- Define data quality metrics and regularly monitor them.
- Implement automated data validation checks and alerts to identify data quality issues in real-time.
- Establish processes for continuous data improvement.
- Encourage employees and data contributors to report data quality issues and provide feedback.
- Conduct regular data audits to ensure that data quality standards are being met.
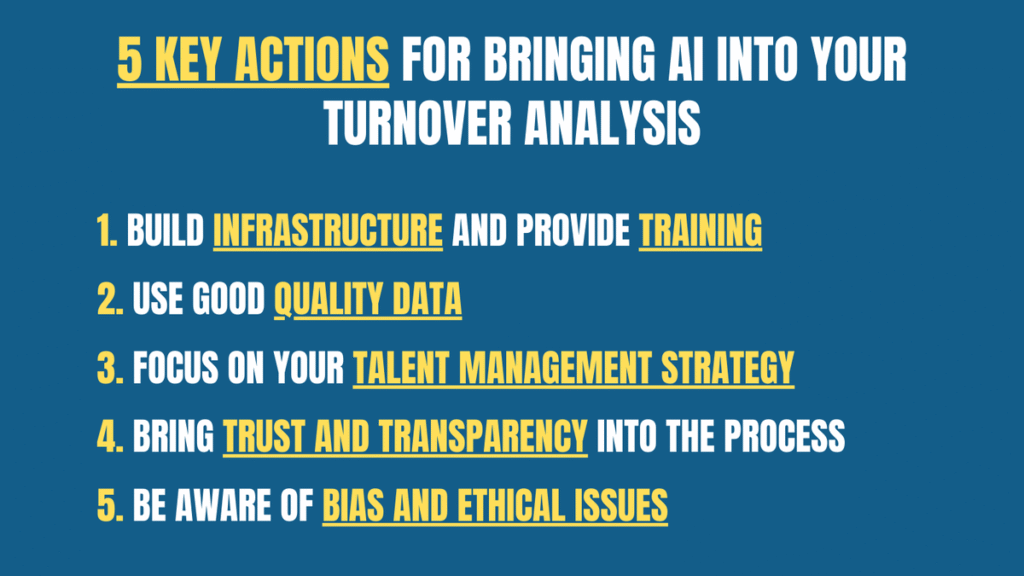
Using AI for managing employee turnover and retention
It’s undeniable that AI-powered people analytics holds immense potential for enhancing your employee turnover analysis. However, if you truly want to embrace all the benefits that this technology offers, you need to embrace it with the right framework in place.
To begin with, you need to foster an AI-driven environment by investing in the appropriate infrastructure and required training. Subsequently, you need to make sure that your data as well as the processes for collecting and processing it are up to good quality and ethical standards.
Finally, you need to use your employee turnover analysis within the context of your broader talent management strategy so you can leverage your retention and engagement initiatives. Only by addressing all the aforementioned factors can you successfully utilize AI to predict employee turnover and retain your most valuable talent.